Data Cleanse & Imputation¶
Introduction¶
Data incompleteness is one of the most common issues in the big data world. Data cleansing and imputation detects empty and invalid fields, then suggests fixes for those fields.
Parameters¶
There are three types of fixes:
Misplaced: This rule applies to each row of the data set to detect rows with invalid values. For example, for a data table with information on apartments, a realistic constraint is that apartments should have at least 1 bathroom. One can define a rule to detect invalid rows, as shown in the below figure. Rows with invalid
number_of_bathrooms
will be detected and fixed with predictions based on other values in the row. Besides concrete values, one can also use regex match to identify the wrong values. The code will automatically surround your input regex with^
and$
to avoid incorrectly matched cells. A resource that may be found useful in constructing and debugging regex expressions may be found here.
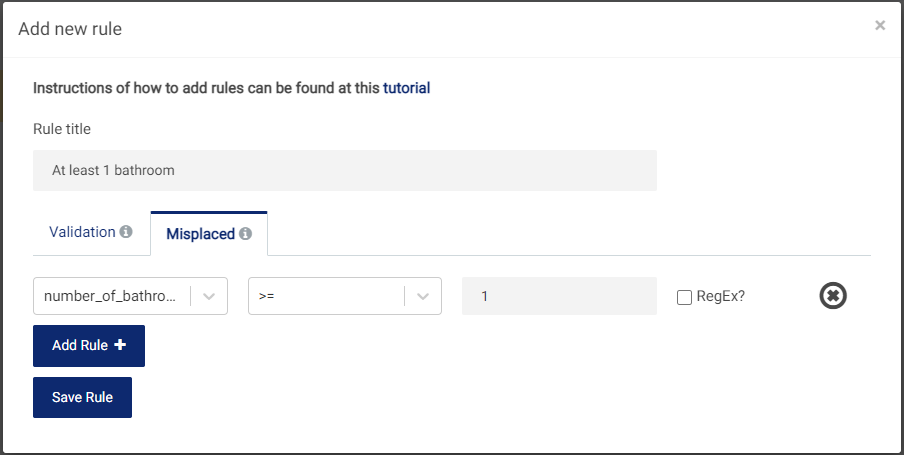
Validation: This rule is applied to rows by comparing fields from different rows. For example, for a data table with information on hospitalisations, hospitals with the same names must have the same phone numbers. One can define a rule to detect invalid rows as shown below, which will be interpreted as the following statement by the algorithm:
when HospitalName equals another HospitalName, if PhoneNumber not equal, either HospitalName or PhoneNumber (or both) is wrong.
The algorithm will try to fix
PhoneNumber
first. However, if it fails to find a value to comply with the criteria, it will try to modifyHospitalName
too. You can put multipleStatement Part
statements, but the algorithm might not work well if there is an insufficient amount of data matched in your dataset.
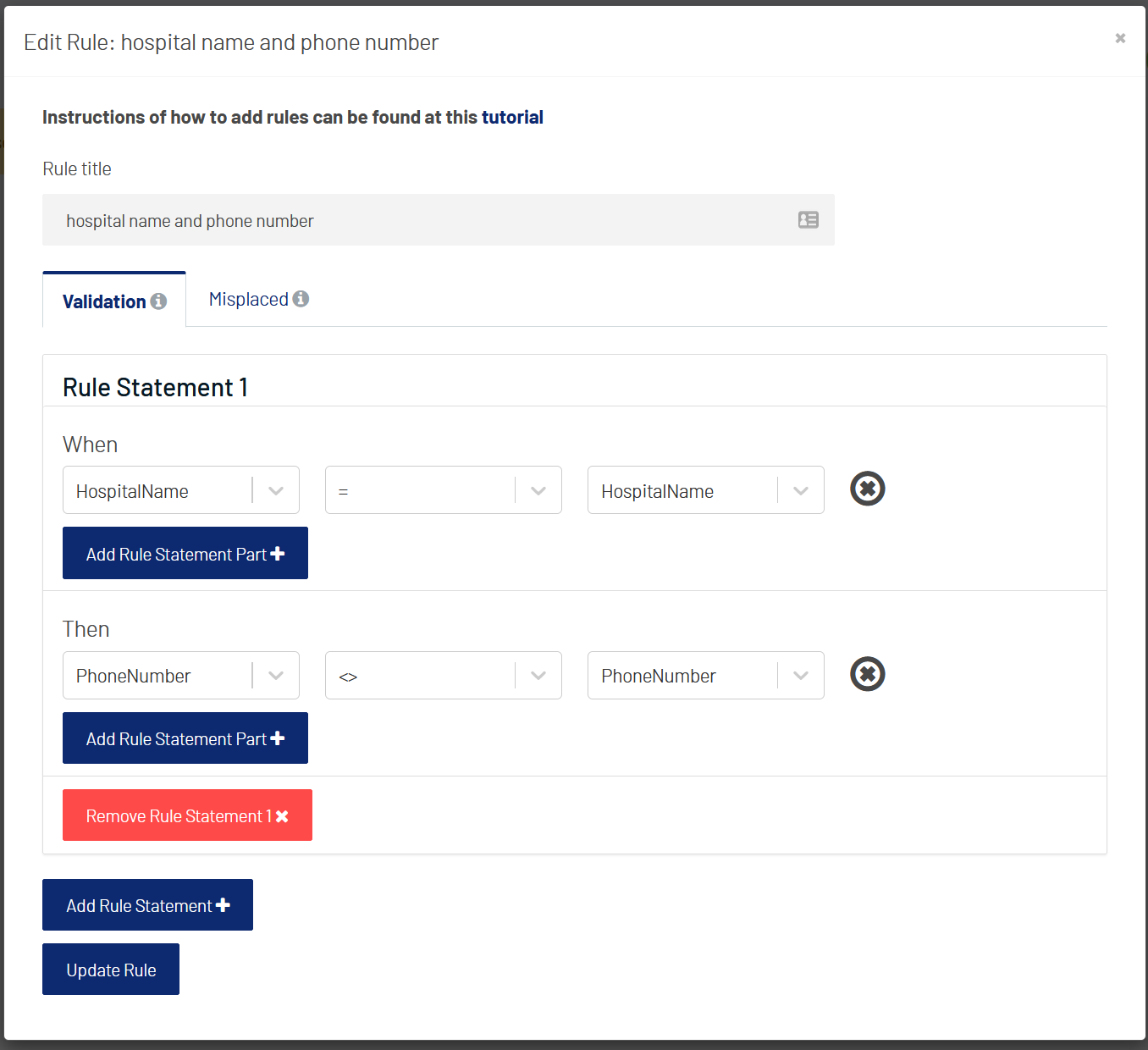
Impute empty or null cells: By selecting this rule, the algorithm will try to generate suggestions for missing values.

If any features are related to time, they can also be used for visualization by setting the following fields:
Time Column: The time-based feature to be used. An arbitrary expression which returns a DATETIME column in the table can also be defined, using format codes as defined here.
Time Range: The time range used for visualization, where the time is based on the Time Column. A number of pre-set values are provided, such as Last day, Last week, Last month, etc. Custom time ranges can also be provided. In any case, all relative times are evaluated on the server using the server’s local time, and all tooltips and placeholder times are expressed in UTC. If either of the start time and/or end time is specified, the time-zone can also be set using the ISO 8601 format.
Case Study¶
Suppose that we have a collection of patient treatment records. However, several typo errors caused the final data to be incorrect. For example, the city of the hospital is supposed to be birmingham
but was typed as birmingxam
, and the phone number for the hospital should be 2565938310
but was recorded as 2x6x938310
.
To fix this problem, we can use the validation rule and set the parameters as shown in the following image. These are based on the knowledge that when the hospital name is the same as the hospital name in another row, their city and phone number should also be the same.
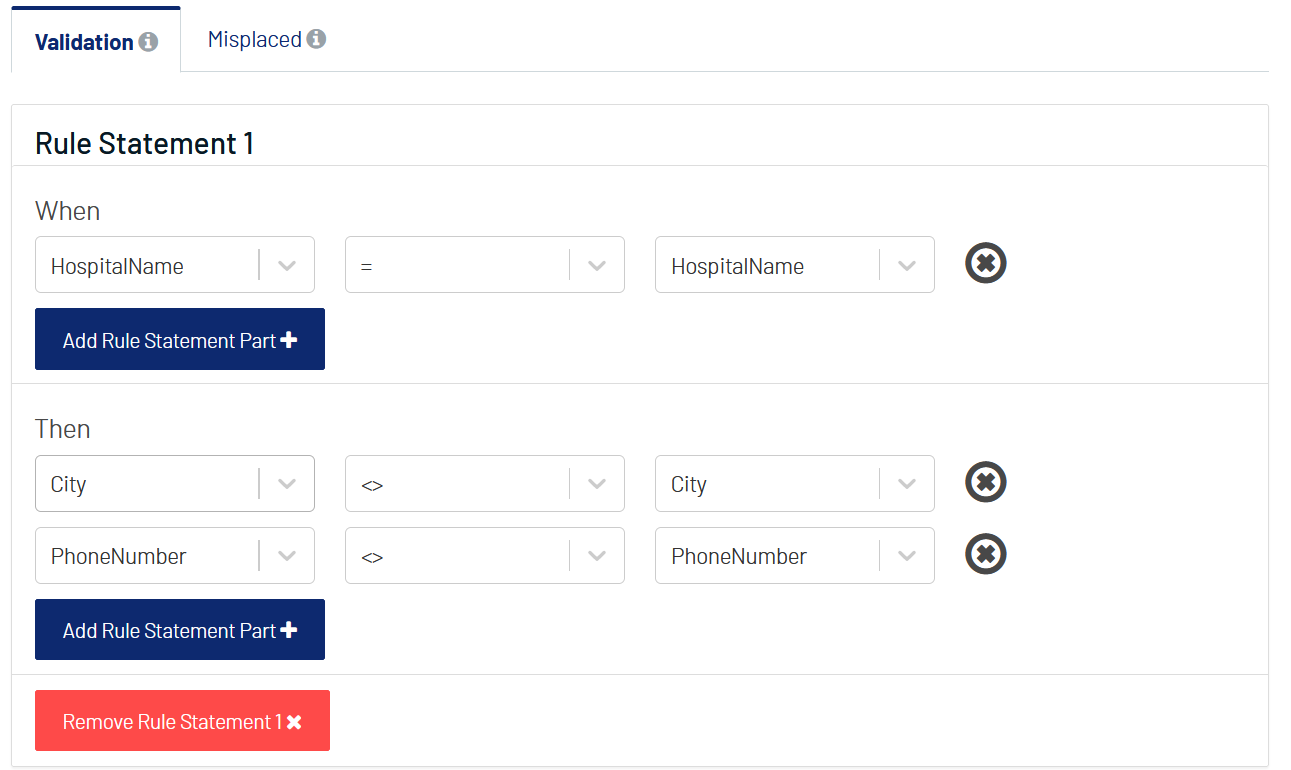
Review Result¶
After running the fix, the fixed data table will be shown with corrected values highlighted in yellow (as in bellow figure). To show the values before correction, one can hover over the cell and the incorrect values will be shown inside brackets in a tooltip. The following example shows that after configuring the validation rule, the algorithm is able to detect the wrong PhoneNumber
and fix the value from 334793870x
to 3347938701
.
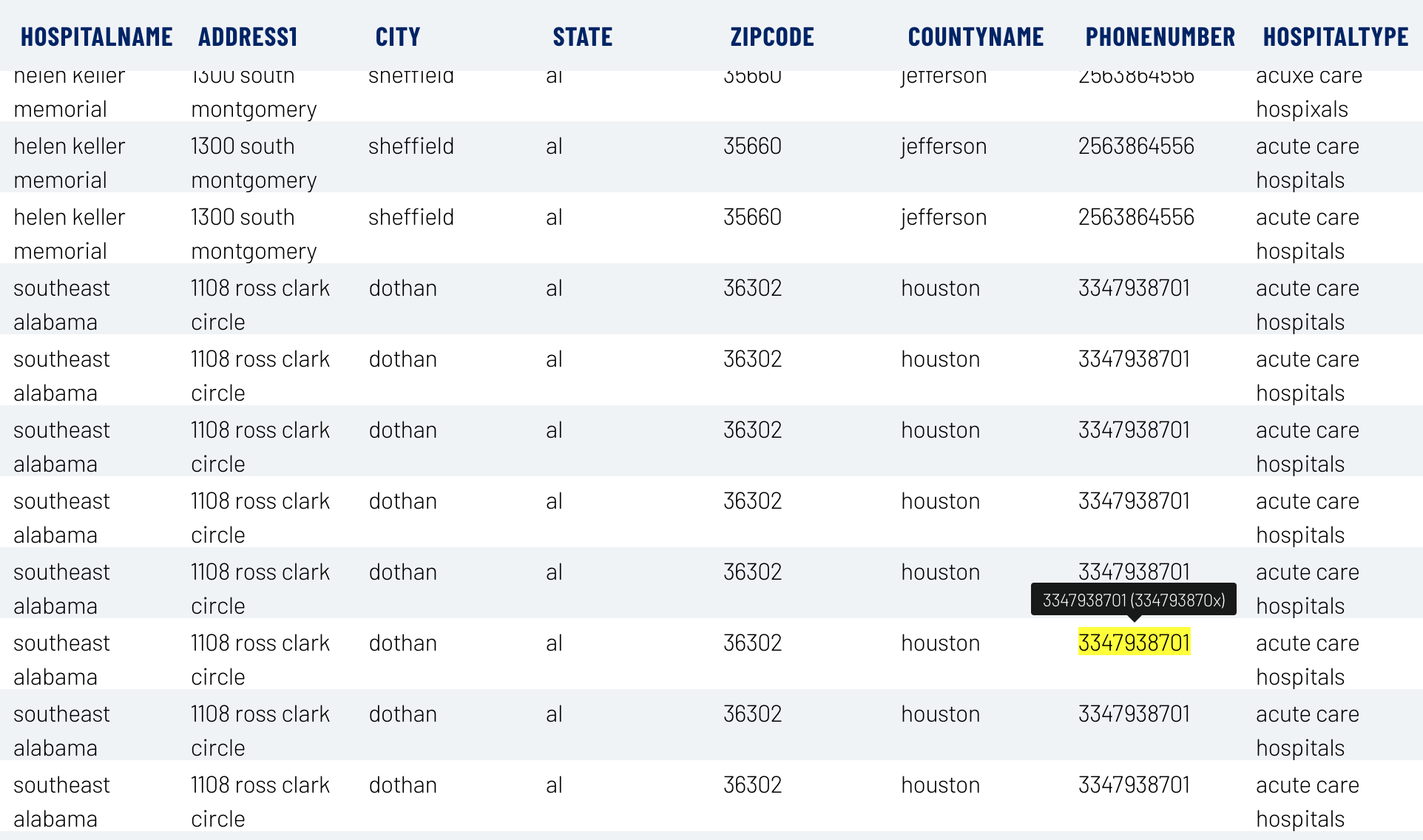